1
/
of
1
Slot Deposit 5000
Slot Deposit 5000 : Link Gacor Slot Depo 5k Atau Slot Min Depo 5rb
Slot Deposit 5000 : Link Gacor Slot Depo 5k Atau Slot Min Depo 5rb
Regular price
Rp 5.000,00 IDR
Regular price
Sale price
Rp 5.000,00 IDR
Unit price
/
per
Link slot deposit 5000 via dana modal receh pasti jackpot, Main di situs slot gacor min depo 5rb dan slot depo 5k pakai akun slot online minimal deposit 5000 tanpa potongan.
Share
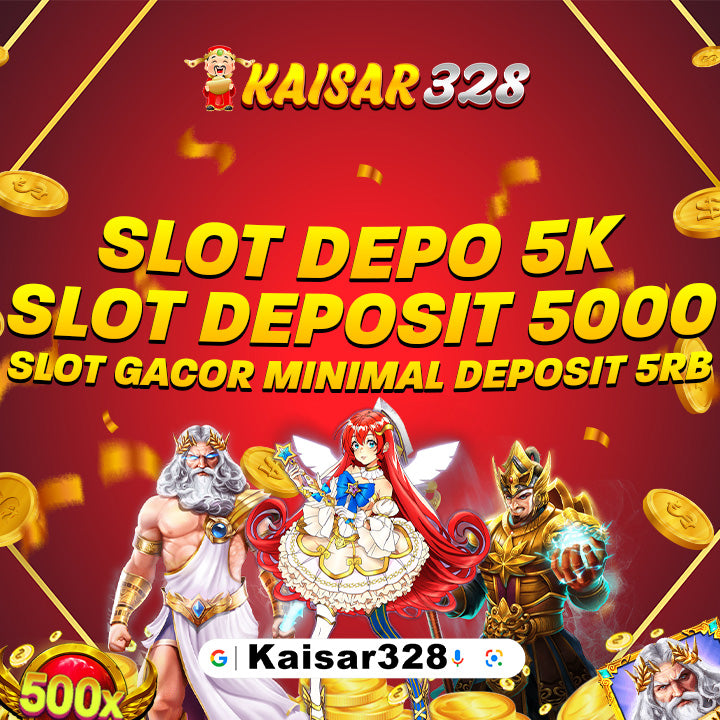